-
Notifications
You must be signed in to change notification settings - Fork 102
New issue
Have a question about this project? Sign up for a free GitHub account to open an issue and contact its maintainers and the community.
By clicking “Sign up for GitHub”, you agree to our terms of service and privacy statement. We’ll occasionally send you account related emails.
Already on GitHub? Sign in to your account
Use Google's project MediaPipe Face Mesh as FACS tracker #33
Comments
Hi, I try the latest |
Let's keep in contact. I'll write up what we have so far. |
Heres a sketch of my approach. I have tooling to bake any vertex cache animation to a skeleton anim, but we need to encode the mesh vertices from mediapipe back into fa units. I think theres is a way. Any suggestions? Like bake permutations of the mediapipe facial mesh from -1 to 1 and manually calculate a key on that track for each face unit |
I am planning to train a neural network to predict each AU intensity or binary classification. Before that, couple preprocessing are required, like centralization and normalization, etc. |
Awesome!
That would be my suggested approach as well. I can't work on this full time, but I can lend you a hand if you would like to? |
Thanks for your offer. I will let you know if I run into any problems. |
I woke up inspired. What do you think about this? Given the tech from Facebook https://github.com/facebookresearch/dlrm or https://ngc.nvidia.com/catalog/resources/nvidia:dlrm_for_pytorch. The input data is mediapipe animations and we label float values for facial units. 468 3D coordinates (landmarks) output of Face Mesh to AU values (1, 2, 4, 5, 6, 7, 9, 10, 12, 14, 15, 17, 20, 23, 25, 26, 28, and 45). So 468 mediapipe floats, 18 facial unit floats and zero categoric features and we label these animation values as true. If we want, we can also add the 52 Perfect Sync floats and VRM facial blend shape floats. The input data would be pairwise values of the floats (mediapipe and perfect sync), (mediapipe and vrm), (media pipe and vrm), and (mediapipe and SRanipal). A third tier would be SRanipal aka Vive facial trackers |
I was researching ludwig-ai for automl approaches. https://github.com/ludwig-ai/ludwig supports tabular, photo (video frames), timeseries and speech samples. Are we able to collect some datasets with labelled data? |
Hey @NumesSanguis , @HarryXD2018 |
I was able to get a standalone data from mediapipe if anyone is still on this project. https://github.com/V-Sekai/mediapipe There's a binary in the github cicd actions for linux and windows. |
Can people provide sample datasets? I can try doing image -> tabular data-> tabular values mappings via ludwig. |
@fire There are plenty public datasets provide AU annotation, like CK+ and DISFA (the dataset used in OpenFace). |
Hi @uriafranko, just to manage expectations, I'm working on other things, so I don't have much time for this project. Others here are working on this topic. |
Hello everyone, just wanted to express my interest and check what kind of help i can provide |
Hi @ymohamed08, thank you for your interest! I'm not familiar with your skillset, so not sure how your help could be best used in relation to this request. Are you into creating ML models? Data engineering? Data validation? Searching the internet for reference implementations? Familiar with FACS? |
I create machine-learning models for emotion detection using facial expressions, I have used Openface so far to do so, but i would like to move to the media pipe. Cannot seem to find a 1-to-1 mapping for some landmarks, or if we can manually create thresholds for each of the action units. Nonetheless, whatever method we use, I would like to be involved and push forwards. |
@ymohamed08 I don't think there is a 1-to-1 mapping between landmarks, because combinations of AUs (Action Units) values do not linearly influence landmarks. You cannot just sum the landmark movement of smiling with the landmark movement of opening your mouth (or any other combination). If you go the route of machine-learning, this is not a problem, because it deals well with non-linear relationships. Get started with a dataset of images that have AU labels. At moment that means certified FACS researchers rate facial configurations on either whether an AU muscle group is contracted or not (boolean value) or also the intensity of the contraction (1-5 with 5 for max contraction). The latter is better for ML, but rating intensity is much more labour intensive, so there is likely less data available. To get started in finding datasets, you can look at the papers by OpenFace: How I would approach this model training: You would run the media pipe's face mesh tracker on all the images/videos in the datasets you collected. Now you have your input data. Scale the AU ratings to 0-1 and that is your target variable. Train a model with face mesh's landmarks as input and AU ratings as output. If you train a model using Python (preferably PyTorch), I can help you turning it into a FACSvatar module, so it can work as a drop-in replacement for OpenFace. The rest of FACSvatar will still work the same then. Hope this can help you get started and let me know if there is anything I can assist/advice on! |
I was able to find this dataset https://www.kaggle.com/datasets/selfishgene/youtube-faces-with-facial-keypoints
|
Overview
As @fire shared in issue 27, Google has released MediaPipe Face Mesh as a Python library (code).
As this solution seems to be fast and is Apache-2.0 Licensed, it's interesting to consider this as a replacement for OpenFace. The tracking output is 468 3D coordinates (x, y, z) for a single mask (1 image or 1 frame in a video), which looks like:
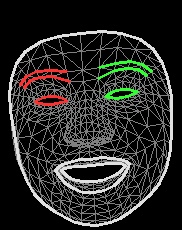
To be useful for this project, the changes in these masks need to be mapped to Action Unit (AU) values according to the Facial Action Coding System (FACS) model.
More info - Demonstration of facial movement per AU.
This can be tricky, because the deformation in the tracking mesh when 2 AUs are active at the same time, won't be always just be adding the deformation of the AUs individually (no linear relation between e.g. AU12+AU14 compared to AU12 and AU14 separately). In practice a linear approach might be good enough though.
A paper by OpenFace on how they did Facial Action Unit detection:
MediaPipe Face Mesh output
A single face mesh tracking a single person in 1 image/frame has 468*3 values. A single landmark:
Note that this output is normalized between [-1, 1].
Full landmark output single mesh (CLICK ME)
Tracking caveats
As of v0.8.7 of Face Mesh, it seems they don't support asymmetric facial expressions (raising only 1 eyebrow or only the right corner of your lips).
Extra info
How to get the landmark coordinates out of the face mesh object: https://stackoverflow.com/questions/67141844/how-do-i-get-the-coordinates-of-face-mash-landmarks-in-mediapipe
Next steps
This might be a research project on itself. Some thoughts:
The text was updated successfully, but these errors were encountered: